Data Mining and Machine Learning
Umar Zai
The Future of Data Mining and Machine learning: Trends to Watch
Data mining and machine learning have revolutionised the way we collect, analyse, and interpret data. With each passing day, these technologies are becoming more sophisticated, leading to new opportunities for businesses and organisations alike. From predicting consumer behaviour to identifying fraud, these technologies have become indispensable for businesses across various industries. However, with a rapidly evolving landscape, it’s essential to stay updated on the latest trends that shape their future. So what does the future hold for data mining and machine learning? In this blog post, we’ll explore some of the latest trends in these fields that you should be watching out for! From AI-driven insights to improved algorithms for predictive modelling, get ready to discover how data mining and machine learning will shape our world in the years ahead.
The potential applications of data mining and machine learning are almost limitless. Businesses can use these tools to gain valuable insights into customer behaviour, develop more efficient processes, and even detect fraud. Machine learning algorithms have been used to improve healthcare outcomes, assist with autonomous driving, and make significant advances in natural language processing. However, the most exciting development lies in the combination of data mining and machine learning technologies for predictive analytics. By combining sophisticated algorithms with large datasets, organisations can better anticipate customer needs and create innovative products that address their evolving requirements.
Data Mining
Data mining is the process of extracting valuable information from large data sets. It is a powerful tool that can be used to identify trends and patterns, and to make predictions about future events.
Data mining is sometimes referred to as “machine learning“, because it relies on algorithms that learn from data. However, data mining is a more specific term that refers to the process of extracting valuable information from data sets.
Machine learning is a broader term that covers a range of techniques for making predictions based on data. Machine learning algorithms are often used in data mining, but they can also be used for other purposes such as detecting fraud or analyzing financial markets.
There are many different types of data mining algorithms, but some of the most popular ones include decision trees, support vector machines, and neural networks.
Every one of these calculations has its own assets and shortcomings, so picking the right calculation for the main job is significant.
Decision trees are good for finding simple patterns in data, but they can be vulnerable to over-fitting. Support vector machines are robust against over-fitting, but they can be difficult to train. Neural networks are very powerful, but they can be hard to understand.
Machine Learning
As machine learning becomes more prevalent, data mining techniques are becoming increasingly important.
Here are some key advancements that are supposed to arise in the field of machine learning including Reasonable simulated intelligence, United Learning, Reinforcement Learning, AutoML, Human-in the know machine learning and GPT-3 and Language Models.
Automated machine learning: This is a trend that is growing in popularity, as it allows for faster and easier development of machine learning models.
Deep learning: This is a subfield of machine learning that is concerned with algorithms that learn from data that is unstructured or unlabeled.
Deep learning has empowered machines to perceive designs in complex information, like pictures and discourse, with amazing exactness. This has prompted forward leaps in applications, for example, picture acknowledgment, normal language handling, and discourse acknowledgment, which were beforehand hard for machines to dominate.
Reinforcement learning: This is an area of machine learning that focuses on how software agents can learn from their environment and improve their performance over time. Reinforcement learning has been utilised in applications like advanced mechanics and gaming with amazing outcomes
Predictive modeling: This is a type of data mining that uses statistical methods to predict future events.
Reasonable simulated intelligence
Reasonable simulated intelligence (XAI) alludes to the method involved with planning and creating AI models that can give clear clarifications of their dynamic cycles. This is significant in light of the fact that many machine learning models, like profound brain organizations, can be hard to decipher, making it trying for people to comprehend how and why the model showed up at a specific choice. XAI is fundamental for building reliable and straightforward computer based intelligence frameworks, particularly in applications like medical services, money, and policing, choices have critical results.
Recent leap in XAI have zeroed in on growing new strategies for deciphering the dynamic cycles of machine learning models. These forward leaps in XAI are probably going to essentially influence the fate of machine learning , making man-made intelligence frameworks more straightforward, reliable, and open to a more extensive crowd.
United Learning can possibly affect different ventures and applications, like medical services, finance, and the Web of Things (IoT). For instance, in medical services, Unified Learning could be utilized to prepare machine learning models on delicate clinical information without disregarding understanding security.
Recent leaps in Unified Learning have zeroed in on working on the proficiency and exactness of the strategy.
Auto machine learning
Auto machine learning alludes to the most common way of computerising the undertakings engaged with building machine learning models, for example, information preprocessing, highlight determination, model choice, and hyperparameter tuning. AutoML plans to diminish the time and aptitude expected to assemble exact AI models.
Auto machine learning is significant on the grounds that it makes AI open to a more extensive crowd, remembering those without particular information for information science or AI. Auto machine learning can likewise assist with speeding up the speed of development via computerizing drawn-out and tedious assignments, permitting information researchers to zero in on additional complicated and imaginative errands.
Human-in the know machine learning
Human-in the know machine learning alludes to a sort of AI framework where people are engaged with the preparation, assessment, and improvement of the model. Human contribution can take various structures, for example, naming information, checking expectations, or giving input on the model’s results. HILML is significant in light of the fact that it tends to the impediments of completely computerised AI frameworks, like predisposition, blunder, and absence of interpretability. Also, by including people in the know, HILML guarantees that the model gains from different and delegate information, is straightforward and logical, and meets the ideal targets and moral guidelines.
The future of data mining and machine learning looks very promising. With the advent of big data, there is a lot of potential for these technologies to grow and evolve.
1. Increased use of artificial intelligence (AI) and machine learning.
2. More sophisticated and accurate predictive models.
3. More use of streaming data (real-time data) for analysis.
4. Greater focus on explainability and transparency of algorithms.
5. Better integration of machine learning with business processes.
The future of data mining lies in combining these different approaches into a single framework that can find complex patterns in data sets. This will require advances in both machine learning and artificial intelligence.
Data mining and machine learning are two of the most popular topics in the field of artificial intelligence (AI). While there is a lot of hype around AI, data mining and machine learning are two areas that are actually delivering results. We will take a look at the future of data mining and machine learning.
First, data mining is expected to become even more important in the coming years. Companies and organisations will continue to collect large amounts of data and use it to drive their decision making. Automated tools such as machine learning algorithms will help them make sense of all this data and uncover hidden trends and patterns.
Second, we can expect machine learning algorithms to become even more powerful. As new algorithms are created and existing ones are refined, they will be able to process larger datasets faster than ever before. Additionally, advanced techniques such as deep learning will enable machines to make more complex decisions based on the data they are presented with.
Finally, there is a lot of potential for combining data mining and machine learning in order to create even smarter AI applications. By bringing together the two fields, developers could create AI-driven programs that can analyse large datasets quickly and accurately in order to uncover useful insights or generate recommendations.
Overall, the future of data mining and machine learning looks bright. As these technologies continue to evolve and become more sophisticated, they will be used by a wide range of industries in order to improve efficiency and make better decisions.
There is no question that data mining and machine learning will keep on filling in ubiquity. The amount of data that is being generated is increasing exponentially, and there is a need for methods to extract useful information from this data. Data mining and machine learning are well suited to this task.
One trend that we see in data mining and machine learning is an increasing focus on explainability. There is a growing realisation that it is not enough to simply produce results – the results must be understandable by humans. This trend is driven by the fact that many important decisions are now being made based on automated systems, and it is crucial that we have confidence in these systems. Explainability will also be important for regulatory compliance – if a system cannot be explained, then it is very difficult to assess whether it complies with regulations.
Another trend that we see is an increasing use of transfer learning. Machine learning models can take a long time to train, so it makes sense to reuse models that have already been trained on similar tasks. Transfer learning can also help when there is a shortage of training data – for example, when you want to build a model for a new
Some trends to watch
1. The increasing popularity of big data is one trend that is sure to continue in the field of data mining and machine learning. With more and more businesses collecting large amounts of data, there is a greater need for effective methods of managing and analysing this information.
2. Another trend that is likely to continue is the use of artificial intelligence (AI) techniques in data mining and machine learning. AI can be used to improve the accuracy of predictive models and to automate the process of building these models.
3. A third trend that is worth watching is the increasing use of cloud-based services for data mining and machine learning. Cloud computing provides a convenient way to access powerful computing resources on demand, which can be helpful for businesses that don’t have their own in-house IT staff.
4. Finally, it’s also worth keeping an eye on the growing interest in open source software for data mining and machine learning. Many popular tools and libraries are available under open source licenses, which makes them more affordable and accessible to a wider range of users.
Conclusion
Data mining and machine learning are rapidly evolving technologies that have the potential to revolutionise many industries. In order to take advantage of this, companies must stay ahead of the curve by understanding the trends and keeping abreast of developments in this field. By doing so, they can ensure that their data mining and machine learning implementations remain relevant and effective for years to come. With advancements in computing power, artificial intelligence, natural language processing, and more, it’s an exciting time for data science enthusiasts who want to keep up with these ever-evolving innovations!
About Remote IT Professional
Remote IT Professionals is devoted to helping remote IT professionals improve their working conditions and career prospects.
We are a virtual company that specializes in remote IT solutions. Our clients are small businesses, mid-sized businesses, and large organizations. We have the resources to help you succeed. Contact us for your IT needs. We are at your service 24/7.
Posted on: April 6, 2023 at 8:27 pm
Best Website Design Companies Houston, Texas
Umar Zai  November 22, 2023
Profiles and Demonstrated Record: Best Website Design Companies in Houston, Texas Houston, Texas, stands as a burgeoning hub for innovation…
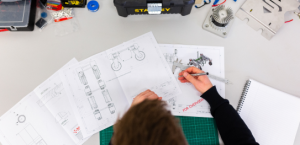
Best Web Design Companies in El Paso
Umar Zai  
Leading in the List: Best Web Design Companies in El Paso, Texas. El Paso is a vibrant city known for…
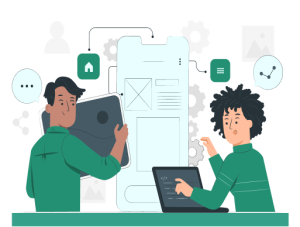
Website Designers San Antonio
Umar Zai  
Ultimate Selection: Best Website Designers in San Antonio, Texas The best website designers in San Antonio, Texas, are highly esteemed…
Cloud Computing Startup Companies
Umar Zai  November 13, 2023
Exploring the Landscape of Popular Cloud Computing Startup Companies Cloud computing has revolutionised the way businesses operate, providing scalable and…
WordPress Blog PlugIns
Umar Zai  
Exploring the best WordPress blog plugins for maximum impact In the dynamic world of blogging, the choice of the best…
AI Language Models
Umar Zai  
Exploring Progress and Obstacles: Delving into the Influence of AI Language Models on Society In the ever-evolving landscape of artificial…
Latest Tweet
No tweets found.