Data Science Trends
Umar Zai
“The Data-Driven Revolution: How Data Science is transforming Our World”
Data Science and its Roots
Data science has its roots in statistics and computer science, with the first use of the term “data science” dating back to the 1960s, when John W. Tukey coined the term in his paper “The Future of Data Analysis.” At this time, data analysis was primarily performed by statisticians, who used techniques such as regression analysis and hypothesis testing to analyze data.
In the 1970s, with the advent of computers, statisticians began using computers to analyze data. This led to the development of the field of computational statistics, which combined statistics and computer science to develop new methods for analyzing data.
In the 1980s and 1990s, with the rise of personal computers and the internet, data became more widely available, and companies began collecting more data than ever before. This led to the development of new tools and techniques for managing and analyzing large datasets, such as data warehouses and business intelligence tools.
In the 2000s, with the rise of social media and mobile devices, data exploded in both volume and variety. This led to the development of new technologies for storing and processing large amounts of data, such as Hadoop and Spark.
In recent years, advances in machine learning and deep learning have led to breakthroughs in areas such as image recognition, natural language processing, and speech recognition. These techniques have been applied to a wide range of fields, from healthcare to finance to marketing.
Overall, data science has a long history, dating back to the 1960s, but it has experienced explosive growth in recent years due to the explosion of data and advances in computing power. The field has evolved to incorporate new technologies and techniques, and is likely to continue to grow in importance as the amount of data generated by humans continues to increase.
Shaping the industry via Data Science Trends
We will now dive into the trends in data science and how they are being applied in real-world scenarios:
- Rise of big data: With the explosion of data in recent years, companies are collecting more data than ever before. This has led to the development of new tools and techniques for analyzing large datasets, such as Hadoop and Spark. For example, Walmart uses Hadoop to process and analyze data from its more than 200 million weekly customers. By analyzing this data, Walmart can optimize its supply chain, improve customer experience, and increase sales.
- Machine learning: Machine learning is a subfield of data science that uses algorithms to learn from data and make predictions or decisions. In recent years, advances in machine learning have led to breakthroughs in areas such as image recognition, natural language processing, and speech recognition. For example, Google’s DeepMind used machine learning to develop AlphaGo, a computer program that beat the world champion at the game of Go.
- Deep learning: Deep learning is a subset of machine learning that uses neural networks with multiple layers to learn from data. Deep learning has led to significant advances in areas such as computer vision, natural language processing, and autonomous vehicles. For example, Tesla’s self-driving cars use deep learning to analyze data from cameras, radar, and other sensors to detect objects on the road and make decisions about how to drive.
- Data visualization: Data visualization is an important part of data science, as it helps to communicate insights and findings to non-technical stakeholders. In recent years, there has been a focus on developing new tools and techniques for visualizing data, such as interactive dashboards and augmented reality. For example, GE Aviation uses augmented reality to visualize data from aircraft engines, allowing engineers to identify potential problems before they become major issues.
- Ethics and privacy: With the growing importance of data in business and society, there has been an increased focus on the ethical and privacy implications of data science. Issues such as bias in algorithms, data privacy, and the responsible use of data have become increasingly important in recent years. For example, Facebook faced criticism for allowing Cambridge Analytica to access user data without consent, leading to increased scrutiny of data privacy practices and the development of new regulations such as the GDPR.
- Artificial Intelligence (AI) Ethics: As AI technologies become more prevalent and powerful, there is a growing concern about their impact on society. Data scientists are now exploring ethical considerations in the development and deployment of AI systems, including issues of fairness, accountability, and transparency. For example, the AI Now Institute has been advocating for ethical and social implications of AI technologies.
- Explainable AI: As AI systems become more complex and opaque, there is a need to understand how they are making decisions. Explainable AI is a growing area of research that aims to develop AI systems that can explain their reasoning to humans. For example, Google’s TCAV (Testing with Concept Activation Vectors) is a framework that allows developers to examine the “thought process” of a neural network and understand how it arrived at its decisions.
- Natural Language Processing (NLP): NLP is an area of AI that focuses on understanding and processing human language. With the explosion of text data on the internet, NLP has become increasingly important in areas such as sentiment analysis, chatbots, and virtual assistants. For example, Amazon’s Alexa and Apple’s Siri use NLP to understand and respond to voice commands.
- Internet of Things (IoT): IoT refers to the interconnected network of physical devices, vehicles, buildings, and other objects that are embedded with sensors, software, and connectivity. This has led to an explosion of data from these devices, which can be analyzed to improve efficiency and productivity. For example, sensors on manufacturing equipment can be used to predict when maintenance is needed, reducing downtime and improving productivity.
- DataOps: DataOps is a methodology that applies the principles of DevOps to data science, with a focus on automating and streamlining the data science workflow. This involves using tools such as version control, continuous integration, and automated testing to improve collaboration and efficiency in data science teams. For example, Airbnb uses DataOps to improve the efficiency and scalability of its data science projects.
- Data Democratization: Data democratization refers to the idea of making data more accessible and available to a wider audience, including non technical stakeholders. This involves developing tools and interfaces that allow users to interact with data and generate insights without needing technical expertise. For example, Tableau is a popular data visualization tool that allows non-technical users to create interactive dashboards and visualizations from data.
Companies Actively Engaged with Data Science
There are many companies across a range of industries that are involved with data science. Here are some examples:
- Amazon –
Amazon employs data science to power its recommendation engines, personalize the customer experience, and optimize supply chain and logistics.
- Google –
Google, another tech giant, uses data science to improve search algorithms, develop self-driving cars, and enhance the user experience for products like Google Maps and Google Assistant.
- IBM –
To develop AI systems, IBM makes use of data science to improve cybersecurity, and analyze large amounts of data in fields such as healthcare and finance.
- Uber –
Data science assists Uber to optimize its ride-hailing platform, predict demand, and improve driver and passenger experiences.
- Airbnb –
With the help of data science, Airbnb analyzes customer behavior, optimize pricing and search algorithms, and identify opportunities for growth and expansion.
- Netflix –
Netflix also uses data science to personalize recommendations, develop original content, and optimize streaming performance.
- Facebook –
Data science is employed by Facebook to power its news feed algorithm, target advertising, and improve user engagement.
- Tesla –
Data science is an important tool for Tesla to develop self-driving cars, optimize battery performance, and improve vehicle safety.
- Microsoft –
Microsoft also uses data science to develop AI systems, improve product performance, and optimize business operations.
- Procter & Gamble –
Another giant that engages with data science to analyze customer behavior, optimize supply chain and logistics, and improve product development and marketing strategies
Challenges facing data science
Despite the many opportunities and benefits that data science offers, there are several challenges that the field faces. Here are some of the key challenges facing data science:
- Data Quality: Data scientists rely on high-quality data to build accurate and effective models. However, data can be messy, incomplete, or biased, which can lead to inaccurate insights and predictions. Ensuring data quality requires careful attention to data cleaning, preprocessing, and validation.
- Data Security and Privacy: Data breaches and cyberattacks have become increasingly common, putting sensitive data at risk. Data scientists must be mindful of data security and privacy concerns, and ensure that appropriate measures are in place to protect data from unauthorized access.
- Interpretability and Explainability: As AI and machine learning models become more complex, it can be difficult to understand how they are making decisions. This lack of interpretability and explainability can make it challenging to trust and use these models effectively.
- Data Bias: Data can reflect historical biases and inequalities, leading to biased models and predictions. Data scientists must be aware of potential biases in their data and models and take steps to mitigate them.
- Talent Shortage: There is a growing demand for skilled data scientists, but a shortage of qualified candidates to fill these roles. This talent shortage can make it challenging for organizations to build effective data science teams and achieve their data-related goals.
- Data Integration: Organizations often have data spread across multiple systems and sources, making it challenging to integrate and analyze this data effectively. Ensuring data integration requires careful attention to data architecture, governance, and management.
- Rapidly Evolving Technology: The field of data science is constantly evolving, with new tools, techniques, and technologies emerging all the time. Staying up-to-date with the latest developments requires continuous learning and adaptation.
Addressing these challenges requires a multi-disciplinary approach that involves not just data scientists, but also experts in fields such as ethics, privacy, security, and governance.
The Future of Data Science
Data science has come a long way since its inception in the 1960s, and it continues to evolve and transform the way we understand and make decisions based on data. However, with great power comes great responsibility, and there are significant challenges that must be addressed to ensure that data science is used ethically and effectively.
As we look towards the future of data science, it’s important to consider how we can ensure that it benefits society as a whole. How can we ensure that data science is used to address important societal challenges, such as climate change or healthcare access? How can we address issues such as data bias and privacy concerns to build trust in data science? And how can we ensure that there is a diverse and inclusive community of data scientists to drive innovation and progress in the field?
These are questions that we must grapple with as we move forward. The answers will require collaboration and innovation from diverse stakeholders, including data scientists, policymakers, and the broader public. By working together, we can ensure that data science continues to be a force for good in the world!
About Remote IT Professionals
Remote IT Professionals is devoted to helping remote IT professionals improve their working conditions and career prospects.
We are a virtual company that specializes in remote IT solutions. Our clients are small businesses, mid-sized businesses, and large organizations. We have the resources to help you succeed. Contact us for your IT needs. We are at your service 24/7.
Posted on: March 5, 2023 at 5:36 pm
Best Website Design Companies Houston, Texas
Umar Zai  November 22, 2023
Profiles and Demonstrated Record: Best Website Design Companies in Houston, Texas Houston, Texas, stands as a burgeoning hub for innovation…
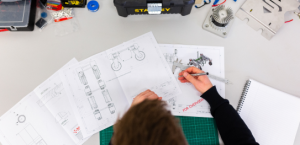
Best Web Design Companies in El Paso
Umar Zai  
Leading in the List: Best Web Design Companies in El Paso, Texas. El Paso is a vibrant city known for…
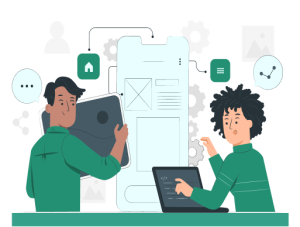
Website Designers San Antonio
Umar Zai  
Ultimate Selection: Best Website Designers in San Antonio, Texas The best website designers in San Antonio, Texas, are highly esteemed…
Cloud Computing Startup Companies
Umar Zai  November 13, 2023
Exploring the Landscape of Popular Cloud Computing Startup Companies Cloud computing has revolutionised the way businesses operate, providing scalable and…
WordPress Blog PlugIns
Umar Zai  
Exploring the best WordPress blog plugins for maximum impact In the dynamic world of blogging, the choice of the best…
AI Language Models
Umar Zai  
Exploring Progress and Obstacles: Delving into the Influence of AI Language Models on Society In the ever-evolving landscape of artificial…
Latest Tweet
No tweets found.