
Generative AI
Umar Zai
Exploring Five Fundamental Concepts of Generative AI: Understanding Bias, GANs, Language Generation, Applications in Healthcare, and Ethical Considerations
Generative AI has emerged as a fascinating field of artificial intelligence, enabling machines to create content, art, and data with human-like creativity. It has rapidly evolved and showcased impressive capabilities in various domains, including image synthesis, language generation, music composition, and more. In this article, we will delve into five fundamental concepts of generative AI: understanding bias, generative adversarial networks (GANs), Language Generation, Applications in Healthcare, and Ethical Considerations. These concepts are crucial to grasp as they shape the development and deployment of generativeAI technologies in diverse industries. Let’s embark on this insightful journey to unravel the essence of generativeAI.
Understanding Bias in Generative AI
Generative AI models gain from tremendous datasets, and if these datasets convey intrinsic predispositions, the models can coincidentally propagate them.
Bias in generativeAI refers to the skewed representation of certain groups or perspectives in the generated outputs. This phenomenon can occur in image synthesis, language generation, or any other creative process.
To address bias, data pre-processing and augmentation techniques are essential. By carefully curating datasets and diversifying training samples, developers can minimise biases in generative AI models. Additionally, ongoing research and collaboration with ethicists help uncover and rectify biases to ensure the technology remains fair and inclusive.
Generative Adversarial Networks (GANs)
Generative Adversarial Networks (GANs) are a powerful class of generativeAI models introduced by Ian Goodfellow in 2014. GANs consist of two neural networks, the generator and the discriminator, engaged in a competitive process. The generator aims to create realistic data instances, while the discriminator’s objective is to distinguish between real and generated data.
Through this adversarial process, GANs continuously improve the quality of generated content, leading to impressive results in image synthesis, video generation, and sound creation. GANs have revolutionised the field of generative AI, providing a new paradigm for creativity and data generation.
Language Generation in GenerativeAI
Language generation is a pivotal application of generativeAI, enabling machines to produce coherent and contextually relevant text. Techniques like Recurrent Neural Networks (RNNs), Long Short-Term Memory (LSTM) networks, and Transformer models have significantly advanced language generation.
Language models can perform tasks such as text completion, machine translation, and even creative writing. However, they can also face challenges, such as generating plausible yet deceptive content, known as “deepfakes.” Researchers continue to work on refining language generation models to achieve both creative and responsible outputs.
Applications of Generative AI in Healthcare
Generative AI has found promising applications in the healthcare industry, aiding in diagnostics, drug discovery, and personalised medicine. One significant application is medical image synthesis, where generative models can generate realistic medical images to augment limited datasets, thus enhancing diagnostic accuracy.
Moreover, generative AI contributes to drug discovery by generating novel molecular structures that could lead to new pharmaceutical compounds. By analysing vast medical records, generative AI models can also predict patient outcomes and recommend tailored treatment plans. Nonetheless, it is crucial to maintain data privacy and ensure ethical considerations are at the forefront of these healthcare applications.
Ethical Considerations in Generative AI
The rapid advancements in generative AI have raised ethical concerns regarding its potential misuse, such as generating fake news, misinformation, or deepfakes for malicious purposes. Furthermore, issues like data privacy, intellectual property rights, and consent must be carefully addressed to ensure the responsible deployment of generative AI technologies.
Transparency, explainability, and accountability are essential principles for developing ethically generative AI systems. Adhering to guidelines and standards, such as the Fairness, Accountability, and Transparency (FAT) framework, can aid in creating trustworthy and ethically sound generative AI applications.
Addressing Robustness and Generalisation in Generative AI
Robustness and generalisation are essential aspects of any AI system, including generative AI. Robustness refers to the ability of a model to maintain high-quality outputs even when faced with perturbations or variations in the input data. Ensuring robustness is crucial to preventing adversarial attacks that attempt to manipulate the model’s behaviour.
Generalisation, on the other hand, relates to the model’s capability to perform well on unseen data or data from different distributions. For generative AI, this means producing coherent and plausible outputs beyond the training data. Achieving robustness and generalisation often involves techniques like regularisation, transfer learning, and fine-tuning, which contribute to more reliable and versatile generative AI systems.
Human-AI Collaboration: Enhancing Creativity and Innovation
Generative AI has increasingly been used as a tool for human-AI collaboration, where AI augments human creativity rather than replacing it. Creative professionals, artists, musicians, and writers have embraced generative AI as a medium to enhance their creative processes and explore new artistic territories.
Generative AI can generate preliminary design ideas, draught musical compositions, or suggest plotlines for writers, acting as a creative catalyst rather than a replacement for human ingenuity. This collaborative approach empowers human creativity while harnessing the efficiency and innovation of AI, leading to synergistic outcomes in various creative industries.
Responsible Data Collection and Privacy Preservation
Generative AI models heavily rely on extensive datasets to learn and generate outputs. However, ensuring responsible data collection is essential to protecting individual privacy and preventing potential misuse of personal information. As generative AI expands into diverse applications, it becomes paramount to establish guidelines for data collection, storage, and usage.
Privacy preservation techniques, such as differential privacy and federated learning, are gaining traction in generative AI research. These methods enable models to learn from decentralised data sources while preserving data privacy, thereby addressing concerns about data breaches and unauthorised access.
Real-time Generative AI: Challenges and Opportunities
Real-time generative AI refers to the ability of AI models to generate outputs instantaneously, making it valuable in applications like video game development, virtual reality, and interactive media. However, achieving real-time generative AI poses significant challenges, as generating high-quality content within tight time constraints can be computationally demanding.
Researchers are actively exploring techniques like model compression, hardware acceleration, and parallel processing to enable real-time generative AI. As this area continues to evolve, we can expect more interactive and immersive experiences across various digital platforms.
Collaborative AI Governance: Navigating Policy and Regulation
The rapid progress in generativeAI necessitates robust governance frameworks to address societal implications and potential risks. Collaborative AI governance involves the collective efforts of governments, industry stakeholders, researchers, and civil society to develop policy frameworks that strike a balance between promoting innovation and safeguarding public interests.
Creating inclusive and transparent decision-making processes is crucial to shaping the future of generative AI. It is essential to establish guidelines that foster responsible research, data sharing, and ethical considerations while ensuring that the benefits of generative AI are accessible to all and used for the betterment of humanity.
Interdisciplinary Research: Fusion of Generative AI with Other Fields
Generative AI’s versatility and potential for creative expression have led to its integration with various disciplines. Researchers are exploring the fusion of generativeAI with fields like neuroscience, psychology, sociology, and economics. By combining insights from these domains, they aim to better understand human cognition, behaviour, and decision-making processes.
The interdisciplinary approach not only enhances the development of generativeAI models but also provides valuable feedback on their impact on human perception and interactions. Collaborative efforts between AI researchers and experts from different domains can lead to groundbreaking applications and a deeper understanding of how generativeAI can contribute to various aspects of human life.
Federated Generative AI: Scaling Privacy-Preserving Applications
Federated learning is a decentralised methodology that empowers numerous gadgets or substances to cooperatively prepare a generative AI model without sharing crude information midway. This approach is particularly relevant in scenarios where data privacy is of utmost concern, such as in healthcare, finance, and smart city applications.
Federated generative AI extends the privacy-preserving benefits of federated learning to generative models, allowing organisations to pool knowledge while protecting sensitive data. By harnessing the power of distributed computing and secure aggregation, federated generative AI facilitates the creation of robust and accurate models without compromising individual privacy.
Continual Learning: Lifelong Adaptation for Generative AI
Continual learning is a paradigm that enables generativeAI models to adapt and learn from new data over time. As real-world environments are dynamic and constantly evolving, continual learning ensures that generativeAI systems remain up-to-date and relevant.
This approach allows generativeAI models to acquire knowledge from new data without forgetting previously learned information, a challenge known as “catastrophic forgetting.” Continual learning is vital in domains where data distributions change frequently, ensuring generativeAI remains adaptable and capable of generating contextually appropriate outputs.
Multimodal Generative AI: Merging Different Data Types
Multimodal generativeAI deals with the synthesis and manipulation of multiple types of data simultaneously, such as images, text, and audio. By fusing diverse data sources, generativeAI can create more comprehensive and interactive outputs.
Applications of multimodal generativeAI range from generating detailed scene descriptions based on images to adding emotional tones to generated speech. Combining various modalities unlocks new creative opportunities and leads to more engaging and interactive experiences for users.
Future Directions: Empowering Human-AI Co-Creativity
Looking ahead, the future of generativeAI holds immense promise for empowering human-AI co-creativity. As generativeAI models become more advanced and accessible, individuals from all walks of life will have the opportunity to collaborate with AI systems to realise their creative visions.
AI-powered tools will serve as collaborators, providing novel insights, suggesting improvements, and expanding creative boundaries. In the future, generativeAI will be a powerful tool for democratising creativity, allowing everyone to participate in and benefit from the dynamic interplay between human imagination and artificial intelligence.
Generative AI and Climate Change Mitigation
The environmental impact of artificial intelligence, including generativeAI, has become a subject of concern. The computational demands of training large generativeAI models can result in substantial energy consumption and carbon emissions. As the field continues to expand, researchers are actively exploring ways to reduce the carbon footprint of generativeAI and make it more environmentally sustainable.
One methodology is the advancement of energy-effective calculations and equipment improvements. By creating models that require fewer computational resources, the environmental impact of generativeAI can be significantly reduced. Additionally, leveraging renewable energy sources for training and deploying AI models can further mitigate the carbon footprint.
GenerativeAI also offers innovative solutions for climate change mitigation. For example, it can aid in generating realistic simulations to study the impact of climate change on ecosystems, weather patterns, and natural disasters. By providing accurate predictions, generativeAI can support policymakers and scientists in making informed decisions and developing effective strategies for climate adaptation and mitigation.
Conclusion
Generative AI has paved the way for unparalleled creativity and innovation, impacting various industries in significant ways. Understanding bias, leveraging GANs, harnessing language generation, exploring healthcare applications, and adopting ethical considerations are five fundamental concepts that shape the trajectory of generativeAI development.
As generativeAI continues to evolve, it is imperative for researchers, developers, and policymakers to collaborate and prioritise ethical frameworks to maximise its positive impact while mitigating potential risks. By striking the right balance between technological advancements and ethical considerations, we can unlock the full potential of generative AI for the betterment of society.
The world of generativeAI is constantly evolving, offering novel solutions, creative expressions, and transformative applications. By understanding and harnessing concepts like bias mitigation, GANs, language generation, healthcare applications, and ethical considerations, we lay the groundwork for responsible and impactful generativeAI development.
Addressing challenges related to robustness, generalisation, real-time capabilities, and data privacy further enhances the potential of generative AI across various domains. Collaboration between disciplines, federated approaches, continual learning, multimodal fusion, and a future focused on human-AI co-creativity herald exciting possibilities for the field.
As we navigate the ongoing evolution of generativeAI, it is crucial to prioritise ethical guidelines, responsible governance, and equitable access to ensure that generative AI continues to be a force for positive change in society. By fostering innovation, creativity, and responsible development, we can embrace the potential of generativeAI to shape a brighter future for humanity.
About Remote IT Professionals
Remote IT Professionals is devoted to helping remote IT professionals improve their working conditions and career prospects.
We are a virtual company that specializes in remote IT solutions. Our clients are small businesses, mid-sized businesses, and large organizations. We have the resources to help you succeed. Contact us for your IT needs. We are at your service 24/7.
Posted on: August 3, 2023 at 11:50 am
Best Website Design Companies Houston, Texas
Umar Zai  November 22, 2023
Profiles and Demonstrated Record: Best Website Design Companies in Houston, Texas Houston, Texas, stands as a burgeoning hub for innovation…
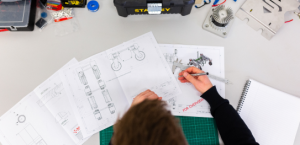
Best Web Design Companies in El Paso
Umar Zai  
Leading in the List: Best Web Design Companies in El Paso, Texas. El Paso is a vibrant city known for…
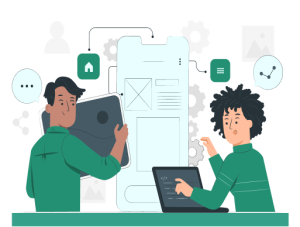
Website Designers San Antonio
Umar Zai  
Ultimate Selection: Best Website Designers in San Antonio, Texas The best website designers in San Antonio, Texas, are highly esteemed…
Cloud Computing Startup Companies
Umar Zai  November 13, 2023
Exploring the Landscape of Popular Cloud Computing Startup Companies Cloud computing has revolutionised the way businesses operate, providing scalable and…
WordPress Blog PlugIns
Umar Zai  
Exploring the best WordPress blog plugins for maximum impact In the dynamic world of blogging, the choice of the best…
AI Language Models
Umar Zai  
Exploring Progress and Obstacles: Delving into the Influence of AI Language Models on Society In the ever-evolving landscape of artificial…
Latest Tweet
No tweets found.